Strategic Signals from the AI Frontier
A Playbook for Leaders Building Leverage in an AI-Native World
The Ventech "AI Impacts & Implications" Summit underscored a profound shift already underway: we are no longer at the beginning of the AI era—we are in its acceleration phase. What matters now is not simply having access to advanced models, but knowing how to translate those capabilities into strategic advantage.
The frontier is rapidly moving from technical novelty to implementation excellence. Competitive edge will go to those who can harness proprietary data, cultivate high-leverage human-AI collaboration, and embed trust and transparency into the fabric of their systems.
Expert perspectives from Dr. Paul Leonardi (UCSB), Heike Schirmer (Yogi), and David Wang (Alpha Design AI) focused squarely on these questions: What happens as AI becomes deeply embedded in daily workflows? What systems must be reimagined? What skills and governance structures will the future demand?
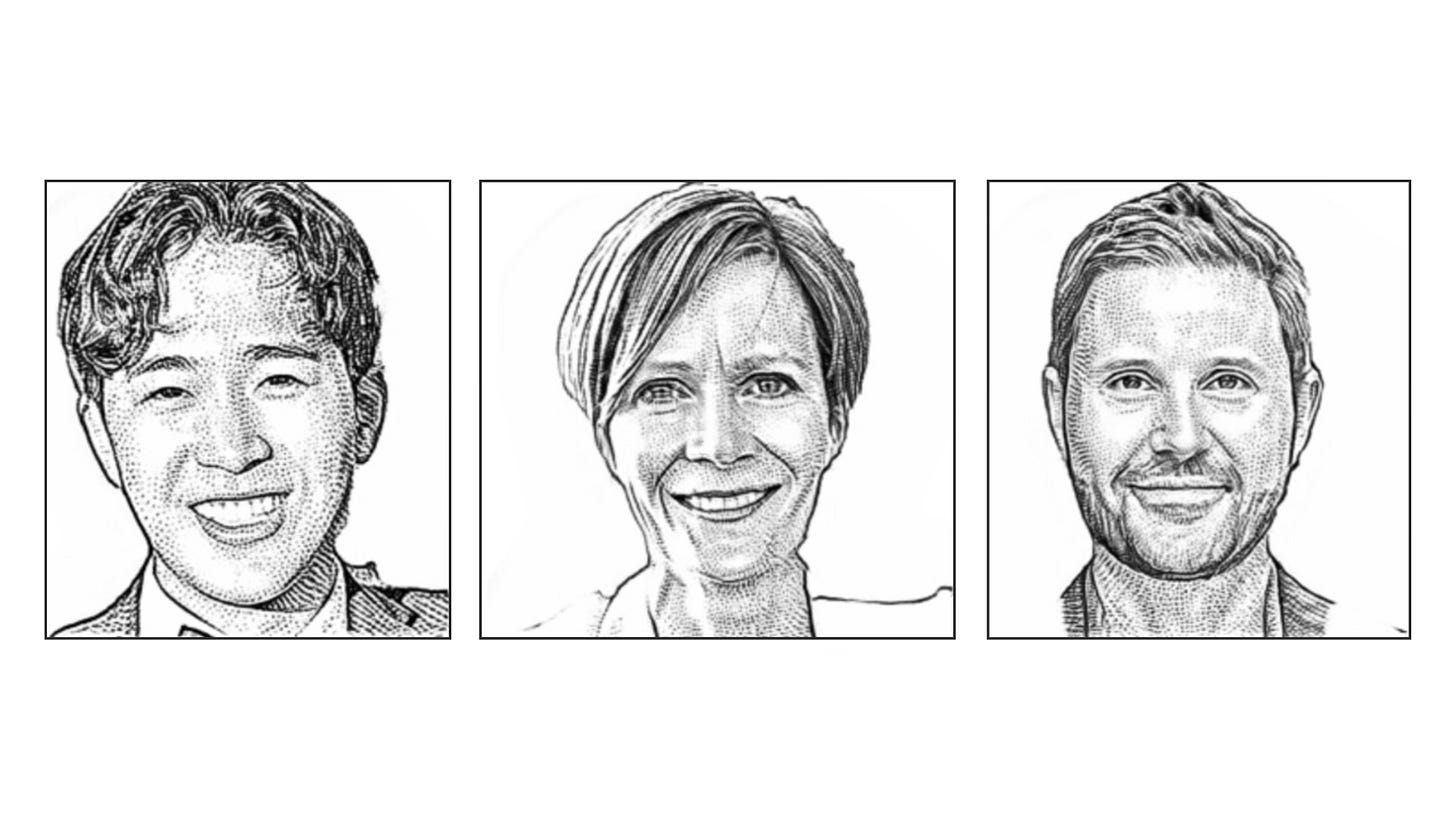
Setting the stage, Schirmer traced today’s AI capabilities back to their symbolic roots—systems like ELIZA in the 1960s that mimicked conversation but lacked true comprehension. Fast forward to 2017, and a real inflection point emerged: the introduction of transformer architectures. As Wang noted, these unlocked the ability to process long-range context and scale training in parallel, setting the foundation for modern generative AI.
But architecture alone wasn't enough. Simultaneously, we witnessed the “digitization of everything”. As Leonardi observed, "Over the last two decades, we've digitized almost every aspect of our world—our locations through apps like Google Maps, our thoughts and ideas on platforms like Facebook and Instagram." This flood of digital information became the fuel for large-scale models, culminating in the ‘ChatGPT moment’ of late 2022 and ushering in a new era—where AI is not just a research topic, but an active force in daily life and business strategy.
5 Strategic Imperatives Emerging from the Summit
From the front lines of AI innovation, five strategic signals are emerging that every leader should have on their radar. These aren’t just trends—they’re tectonic shifts shaping the future of work, competition, and value creation:
Data scarcity will soon become AI's biggest bottleneck — high-quality public datasets could be exhausted between 2026-2032
Human-AI hybrid teams deliver 5x productivity gains — without replacing workers
Agentic AI will transform workflows — moving beyond content generation to taking independent actions
Trust and transparency are becoming competitive advantages — not just compliance checkboxes
Recursive innovation cycles are accelerating — as AI tools begin designing better versions of themselves
Let’s unpack each—and explore how to turn insight into advantage.
From AI-Generated Music to Business Transformation
The Summit opened with something unexpected—an AI-generated barbershop quartet performance that sounded remarkably professional. Prior to the reveal that the song was created using Suno, one audience member suspected something was amiss when they observed the song was "too perfect" and perhaps not humanized enough.
This playful demonstration showcased both AI's impressive capabilities and its occasional unpredictability—contrasting a version representing polished output with another that demonstrated generative AI going off the rails and creating incoherent gibberish.
You can revisit to the songs here:
“Harmonizing Tomorrow: The AI Barbershop Overture” by The Close Shaves, demonstrating professional-sounding AI generated music
“Song Generation Gone Wrong: Suno Outtakes” demonstrating current limitations of AI
This juxtaposition perfectly framed the Summit's central theme: today's AI systems are simultaneously revolutionary and imperfect. As one panelist noted, we're navigating a world of probabilistic models that may be accurate 90-95% of the time, but carry a chance of unexpected behavior.
1. The Coming Data Drought: Your Company's Information Is Becoming Its Most Valuable Asset
While headlines focus on expanding computing power, the panelists identified a different constraint that could soon limit AI progress: high-quality data.
Heike Schirmer of Yogi revealed that public data availability is growing exponentially slower than model capacity needs. Early models like GPT-3 trained on relatively modest datasets, but each subsequent generation required orders of magnitude more data—a trajectory that's becoming unsustainable.
"A study shared by Epoch AI predicts we may exhaust the supply of high-quality public data between 2026 and 2032," Schirmer explained. "We're facing a data drought just as AI capabilities are expanding."
The strategic implications are profound:
Your proprietary data is becoming irreplaceable — Organizations with unique, domain-specific datasets gain powerful leverage that competitors can't replicate
Data literacy is now a critical leadership competency — Understanding what unique data sources your organization possesses and how to leverage them is becoming evermore a strategic skill
Synthetic data carries hidden risks — As Paul Leonardi warned, relying too heavily on synthetic data risks "model collapse," where systems produce increasingly homogeneous, less innovative outputs
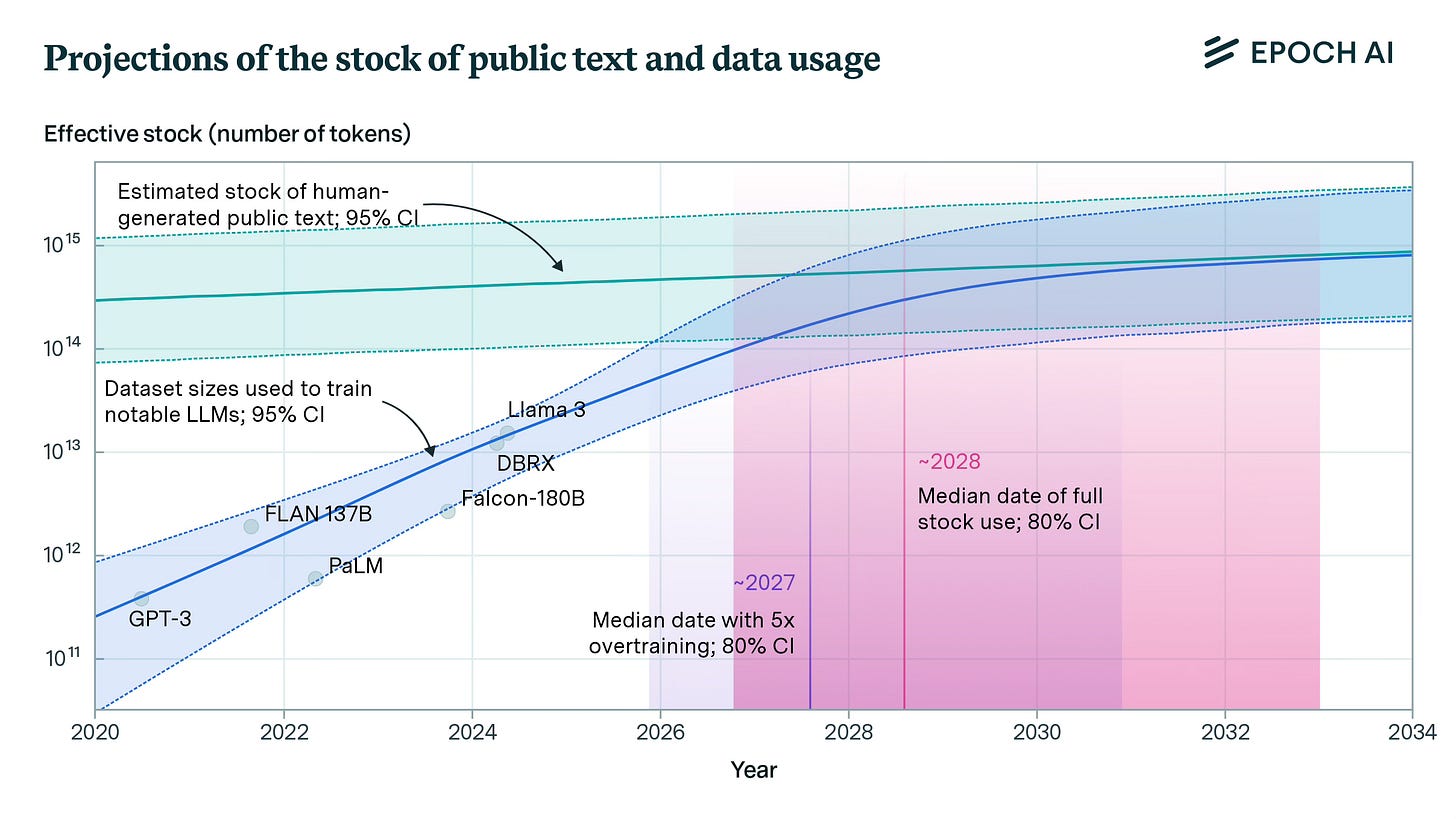
As David Wang summarized: "In a world of abundant models, the rarest resource is reliable, original data."
2. The Human-AI Partnership: Why Collaboration Beats Replacement Every Time
The real story isn’t workforce replacement—it’s workforce amplification. The Summit revealed compelling evidence that the most successful AI implementations aren't replacing humans—they're augmenting them in ways that make both more effective.
Paul Leonardi shared a fascinating case study from a life insurance firm that automated routine underwriting decisions. Instead of eliminating positions, the company worked with underwriters to identify higher-value activities where human judgment remained essential:
"They weren't trained to use AI—but to do the things AI couldn't."
The human underwriters shifted from reviewing routine applications to handling complex edge cases that algorithms struggled with—applications that didn't fit neatly into standard categories but represented valuable business opportunities. What made this transition successful wasn't technical training but investment in communication skills, empathy, and decision-making capabilities.
This pattern is emerging across industries:
Wang cited his own experience at Alpha Design AI where software engineers are now able to review 5x more code with AI assistants
Schirmer shared that her work at Yogi reveals that AI-empowered customer success teams handle larger caseloads while maintaining deeper relationships
Leonardi identified exciting research breakthroughs enabled by combining AI analysis and pattern recognition with human intuition
The key takeaway? Organizations must prepare for effective collaboration between humans and machines, shifts in roles and responsibilities, and the emergence of new positions requiring different skill sets. As AI advances, deeply human capabilities—communication, empathy, strategic thinking—become even more valuable.
3. Trust is the New Interface: Why Explainability Drives Adoption
"It's not just about generating answers—it's about knowing where they came from."
As generative AI becomes ubiquitous, the spotlight has shifted to trust. David Wang highlighted an emerging frontier: retrieval-based generation, where outputs are grounded in verifiable sources rather than opaque model processes.
Paul Leonardi reframed this concept powerfully: governance isn't just compliance—it's part of the user experience of trust.
Leaders in this space are:
Creating ethics councils to address emerging questions
Implementing explainable AI technologies (like SHAP value visualizations)
Maintaining human oversight of critical decisions
Building transparency into their product design
Wang highlighted explainability as a major limitation of current AI systems: "If you type something and get a completely coherent English sentence back, we don't really know what's going on under there, and so that makes it very hard to trust."
Organizations that build transparent, trustworthy AI systems will have a significant advantage in adoption and impact.
4. Beyond Generative AI: The Rise of Agentic Systems
Among the most forward-looking insights from the Summit was the shift from generative AI to agentic AI—systems that don’t just respond to prompts but take independent action on behalf of users.
Heike Schirmer shared a striking example: an early prototype that could navigate her desktop, make restaurant reservations, and carry out multi-step tasks. While still imperfect, it marked a meaningful leap—from AI that outputs content to AI that accomplishes goals with autonomy.
Paul Leonardi added important context: even the simplest tasks often require a cascade of micro-decisions. Building agents that can reliably manage this complexity is both technically demanding and strategically significant.
Leonardi also surfaced the deeper implications:
“Imagine if we come back together in three years and talk about how our AI assistants operate on our behalf. What happens when you've got an agent out there representing you, making decisions, and reflecting you to other people?”
Agentic systems raise fundamental questions about identity, delegation, and trust. As they become more capable, leaders will need to decide not just what these agents can do—but how much authority they should be given to act on our behalf.
5. Recursive Innovation: When AI Starts Designing Its Own Future
Imagine an AI model designing better chips that will, in turn, run even more powerful models. This isn't science fiction—it's happening today at Alpha Design AI, makers of ChipAgents.
David Wang introduced the concept of recursive innovation, where software improves hardware in a continuous feedback loop. This pattern of self-improvement represents a fundamental shift in how we think about technological progress. Rather than linear advancement, we're entering an era of compounding capabilities, where each innovation accelerates the next in unprecedented ways.
This has implications far beyond hardware design:
AI-optimized algorithms that further improve model capabilities
Self-improving data analysis systems that identify their own limitations
Productivity tools that learn to streamline themselves based on usage patterns
The organizations that harness this recursive potential will see compounding advantages that become increasingly difficult for competitors to overcome.
Bold Predictions: Where We're Headed
As the Summit concluded, the panelists offered both optimistic and cautionary predictions about AI's future:
Near-Term Acceleration
Scientific Innovation Will Flourish - Paul Leonardi predicted an explosion of scientific breakthroughs driven by AI's pattern recognition capabilities. Unlike humans, who are limited by their "perceived sense of relevance," AI can identify unexpected connections across domains. Projects like Google's AlphaFold are already demonstrating this potential, with hundreds of AI-generated hypotheses being confirmed in laboratories.
Value Creation Will Accelerate - Heike Schirmer highlighted how AI could dramatically reduce wasteful innovation cycles. In consumer packaged goods, for instance, 90% of new products fail. By combining customer data with AI insights, companies could focus on products with higher probabilities of success, eliminating waste and accelerating time-to-value.
Software Will Become Conversational – David Wang predicted a major shift in how we interact with technology, moving from manual workflows to AI agents that collaborate through natural language. Rather than navigating menus or clicking through interfaces, users will increasingly express intent—and let intelligent agents handle execution. This new interface paradigm could redefine productivity across every professional domain.
Long-Term Concerns
The Homogeneity Risk - Paul Leonardi outlined an undesirable scenario where AI models, trained on increasingly similar data sets, produce increasingly homogeneous outputs. "We're going to get similar calendars... similar thank you notes... similar sounding innovations." This standardization could potentially lead to stagnation in creative and innovative domains if not addressed.
The Limits of Prediction - David Wang offered a humbling reminder about how expectations shape strategy: "When building or investing today, teams operate with an implicit forecast of what technologies will be available, viable, or dominant years down the line. This forecast shapes product roadmaps, hiring plans, infrastructure choices, and strategy." While significant change is inevitable, its specific direction remains uncertain, creating potential blind spots if not regularly examined.
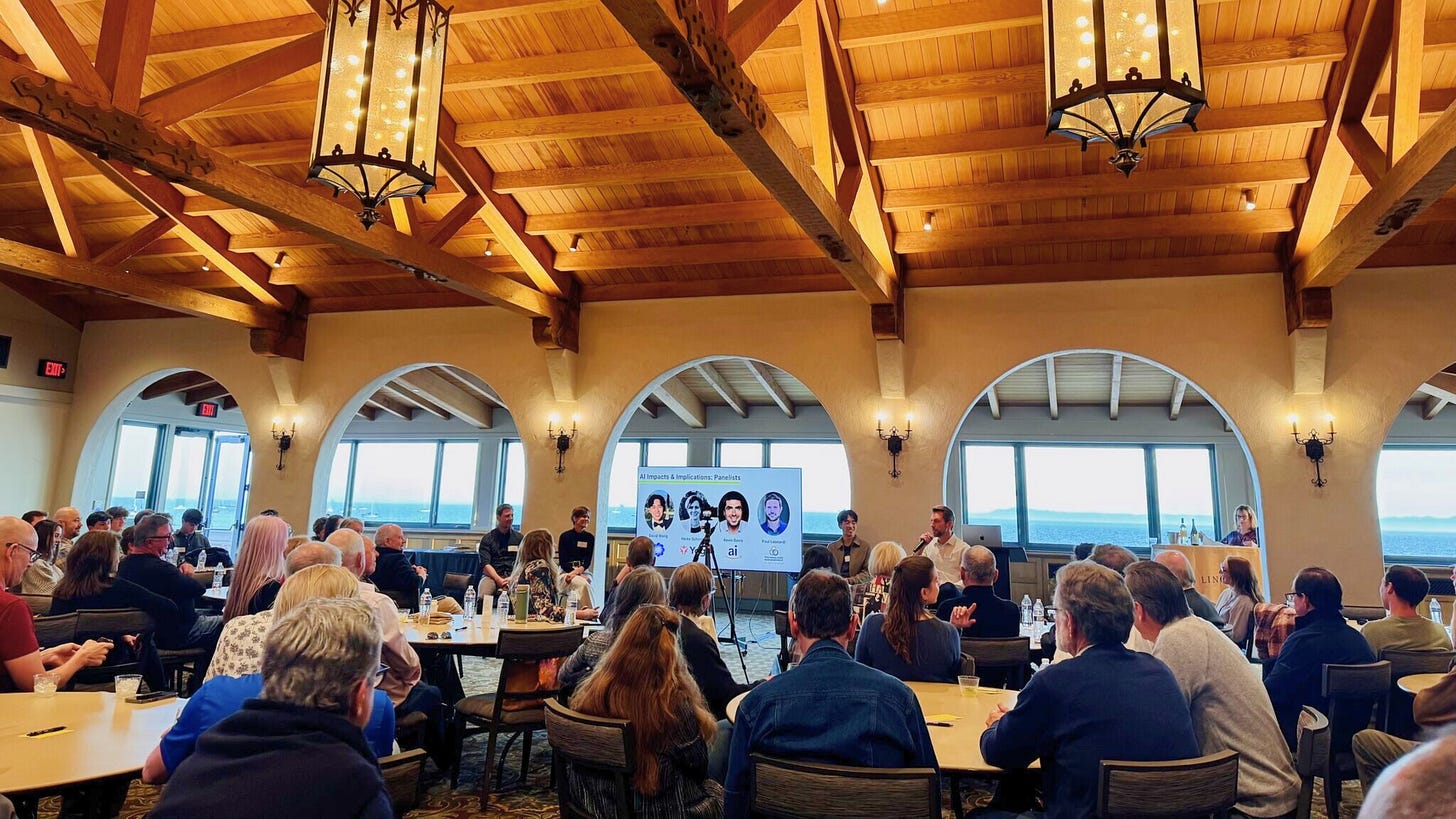
Building Your AI-Ready Organization: A Practical Framework
For leaders wondering how to integrate AI effectively, the panel offered practical guidance based on real-world experience.
1. Start Where Data Is Rich and Processes Are Formulaic
The most successful AI implementations target areas with three crucial characteristics:
Large amounts of available data
Relatively formulaic processes
Potential for identifying emergent patterns
This "sweet spot" often exists in customer service, sales, marketing, software engineering, and R&D—functions that Paul Leonardi suggested will capture 75% of AI's value in the near term.
Action step: Identify 2-3 opportunities with these characteristics and conduct a pilot assessment of AI readiness.
2. Empower Individual Contributors to Experiment
Rather than imposing top-down AI mandates, successful organizations create space for frontline employees to experiment with AI tools in their daily workflows.
As Paul Leonardi observed, "Our predictions about where AI can be most useful seem to almost always be wrong... because the more senior you are at your organization, the less you understand about how people are doing their everyday work."
Action step: Create an "AI sandbox" program where employees can test tools and document productivity improvements.
3. Develop Data Literacy Across All Functions
Statistical literacy and data awareness are becoming essential skills for every role. As Leonardi emphasized, "I don't care if you're in the copywriting function of your organization or you're doing R&D, if you don't understand how a prediction model works, then it's going to be really difficult to be able to interface with tools we have today and definitely the tools that we're going to have in the future."
Action step: Implement basic data literacy training that includes:
Understanding statistical principles like sample size, outliers, distribution patterns, and confidence intervals
Identifying valuable data assets specific to your organization
Recognizing the limitations of AI models
4. Build Trust Through Governance and Transparency
Governance isn't just risk mitigation—it’s the foundation for trust and adoption. As AI systems become more autonomous and influential, embedding transparency and oversight into core operations is no longer optional.
The panel emphasized several key practices:
Establishing clear data governance frameworks that balance innovation with privacy
Implementing explainable AI technologies that reveal how decisions are made
Forming cross-functional ethics councils to address emerging risks
Maintaining human oversight in critical decision-making contexts
Action step: Form an AI governance council with representatives from legal, security, product, data science, and frontline teams. Integrate explainability, risk review, and human oversight into your development lifecycle.
Venture Opportunities on the Horizon
For entrepreneurs and investors, the Summit highlighted several promising opportunity spaces:
Knowledge Management — Paul Leonardi identified capturing organizational knowledge as a major opportunity. "We hire smart people. They learn new things, and we have lots of knowledge internally. It's really difficult to tap into." Systems that can harvest insights from existing communications and deliver them at the moment of need represent a significant opportunity.
Data Infrastructure — David Wang suggested that just as the California gold rush made selling picks and shovels a lucrative business, the "AI gold rush" creates opportunities for companies that help organizations manage, analyze, and leverage their data assets.
Enterprise Operations Optimization — Prior to the panel discussion, the event featured an impressive showcase from Execify, a startup building executive operations tools that use AI to streamline scheduling, communications, and analytics while providing real-time insights into organizational efficiency.
The 5 Imperatives: Your AI Readiness Checklist
As we synthesize the Summit's insights, five clear imperatives emerge for organizations navigating the AI frontier:
Treat data as a strategic asset — Identify, cultivate, and protect the unique data sources that provide competitive advantage.
Build human-AI collaboration models — Focus on upskilling for the capabilities AI can't replicate, while thoughtfully automating formulaic tasks.
Foster a culture of experimentation — Empower teams to discover AI applications from the bottom up, rather than imposing them from the top down.
Develop robust governance frameworks — Balance innovation with trust, transparency, and privacy considerations.
Prepare for agentic capabilities — Begin thinking now about how autonomous AI systems might represent your organization and employees.
The future emerging from these insights isn't one of human replacement, but of unprecedented augmentation—where AI handles repetitive tasks with efficiency while humans focus on judgment, creativity, and connection.
As David Wang succinctly put it: "AI has profoundly changed the way we work... and on both an organizational and individual level, we have to basically work with it."
Heike Schirmer added an important nuance: "With it—and not against it."
Which AI Theme Should We Explore in Greater Depth?
AI Readiness Canvas
Ready to assess where your organization stands?
Drawing from the Summit’s core themes, this AI Readiness Canvas offers a simple way to evaluate your current state—and spark meaningful conversations about where to focus next.
Trust & Explainability
Do our models surface source attribution?
Have we designed governance features that are intuitive and user-friendly?
Data Strategy
What unique datasets do we have?
How are we securing and enriching them?
Team Design
Are we training for complementary human-AI skills?
What roles are emerging—and which are evolving?
Experimentation Culture
Where can we safely prototype AI workflows?
Do we incentivize exploration?
Recursive Thinking
Are we designing tools that learn from and improve one another?
What systems could become self-optimizing?
Share your canvas results in the comments to continue the conversation with the community!
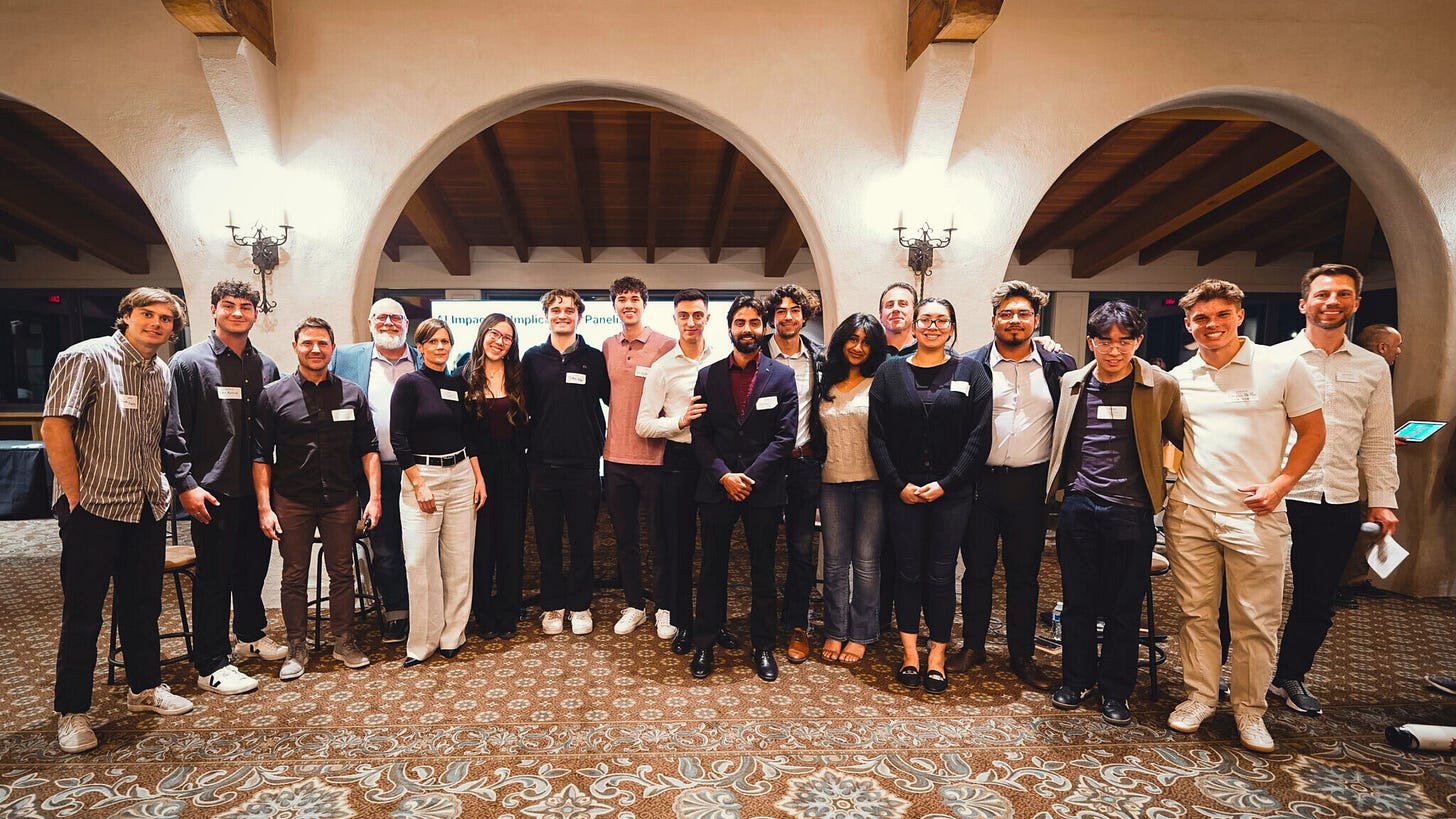
What's Coming Next
In our next post, we’ll explore some of the most compelling audience questions that we didn’t have time to address live—questions that push the conversation forward, including:
“At what point will AI systems begin significantly driving their own evolution?”
“How can organizations prevent model collapse?”
“What techniques can businesses use to optimize data for generative search results?”
“What novel roles or professions might emerge?”
“How should organizations measure ROI beyond simple efficiency gains?”
Subscribe now to get these exclusive insights delivered straight to your inbox!
For more in this series, revisit Multilogue’s coverage of AI at the Inflection Point: Why Ventech's AI Impacts & Implications Event Could Redefine Your Innovation Strategy.
Want to push your creative horizons even further?
Explore ideas, ask bold questions, and prototype your next big thing with MultilogueGPT—our custom AI collaborator designed for curious minds.